Time is relative, especially regarding how a given business organization can handle (and benefit from) its internal data capabilities. Orgs steeped in capital, time, and talent may still be stuck in the data management starting blocks, unable—or unwilling—to foster and leverage high quality data.
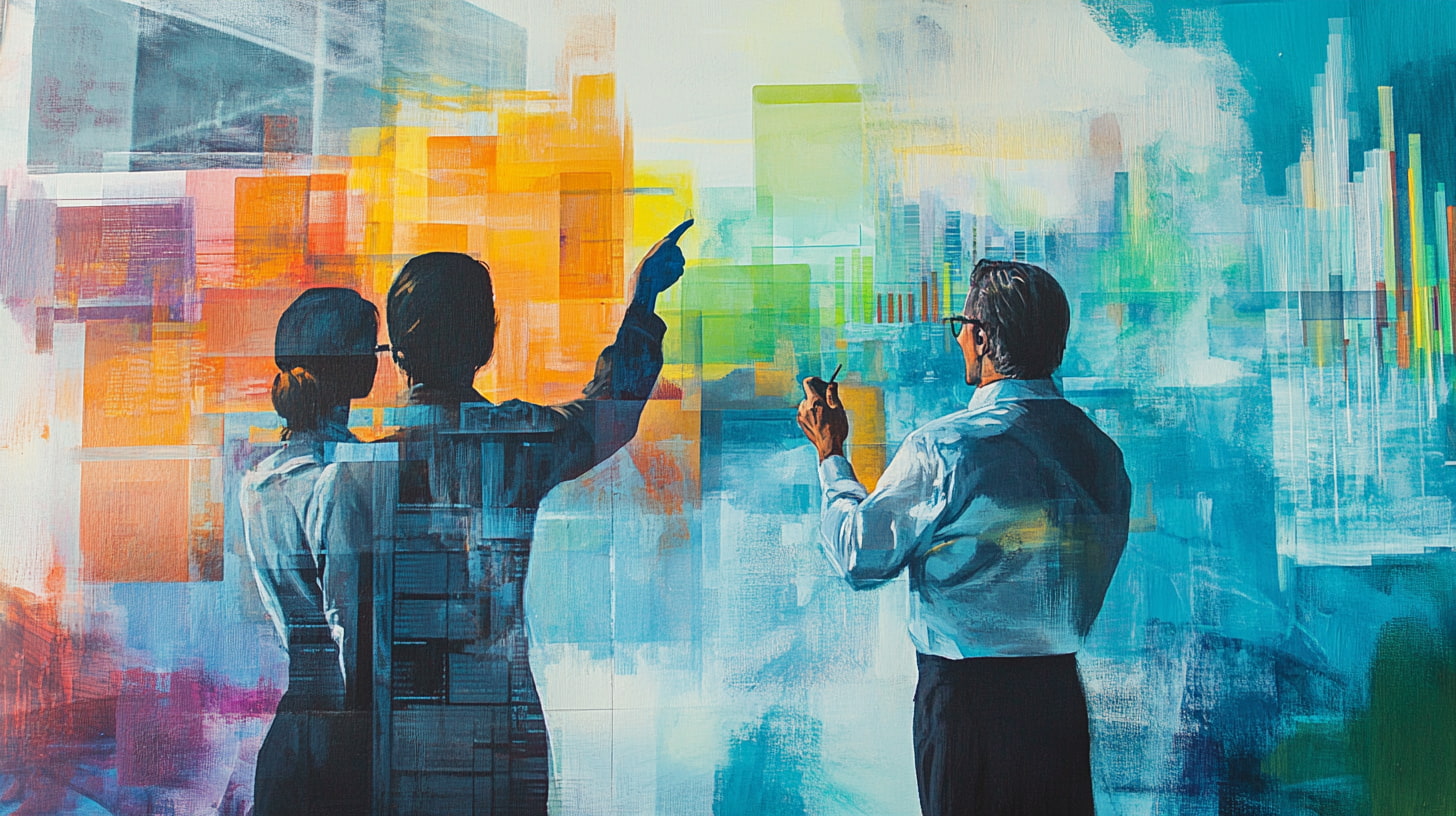
This makes it cruicial for IT leaders to gauge their organization’s data maturity and keep data quality high—and keep overhead, inefficiencies, and data issues low.
Enter: Data maturity modeling.
What are data maturity models?
A data maturity model is a framework that’s used to get a grasp on an organization’s data capabilities and data assets. In doing so, maturity models are key for IT leaders and stakeholders to assess data quality and how well an organization leverages its data. That and, ideally, to improve both.
For data engineering practitioners, data maturity models serve as a roadmap for enhancing data management practices and how they’re applied in actual day-to-day operations.
For the data engineering-adjacent—business leaders, data consumers, stakeholders, etc.—a data maturity model can provide critical insights and strategic guidance for how everyone in the organization can play a role in leveraging data as a valuable asset. This means data maturity models, when realized and fully embraced, work to align data initiatives with business objectives, ensure better decision-making, and promote a culture that treats data as a valuable product.
1. Stages of data maturity
Stages in data maturity models represent a clear linear progression path. They are essential for measuring the journey organizations take as they work to enhance their data capabilities over time.
Leaders within organizations can reference prior stages to maintain a clear handle on the maturation process taken to date while getting a firmer sense of what steps are needed to reach higher levels of data maturity.
Benefits:
- Benchmarking: In addition to keeping their bearings, judicious use of benchmarks enables IT and business leaders to compare their organization’s current capabilities against those of their competitors.
- Goal setting: By assessing the specifics needed to progress to the next stage of a given maturity model, IT leaders can codify clear goals in the digital maturation process. When goals are clearly defined, IT leaders are able to work out how best to move ahead most strategically.
- Roadmap development: By fleshing out a given strategy into an actionable roadmap, leaders can set milestones to measure progress between stages, ensure key roles are clearly defined, identify potential risks, and maintain stakeholder buy-in as the plan unfolds.
2. Assessment areas
Working in conjunction with maturity stages, assessment areas define the specific aspects or domains of data management and data governance that need to be evaluated during the modeling process.
The inclusion of assessment areas ensures that the modeling process will include a comprehensive evaluation of all relevant and critical components of an org’s data maturity.
Benefits:
- Holistic evaluation: Regardless of the specific maturity model used, defining assessment areas ensures assumptions are made regarding all aspects of governance and data management.
- Gap identification: The value of assessment areas is that they can reveal aspects of data governance and management that should be present—not just those that already are. Identifying these gaps as part of a data maturity assessment is instrumental for data leaders needing to produce a cohesive data strategy moving forward.
- Focused improvement: An additional boon to strategic planning, focused improvement areas allow organizations to enact roadmaps that leverage resources efficiently—with efforts spent on the most critical areas in respect to achieving that next data maturity stage.
3. Themes and dimensions
Models incorporate themes and dimensions to provide those measuring an organization’s data maturity with overarching principles to follow and specific criteria for assessment.
As a result, the data modeling process unfolds with a helpful degree of consistency as opposed to ad-hoc approaches that are difficult to replicate.
Benefits:
- Assessment clarity: Themes and dimensions break down the broader concept of data maturity into specific, manageable categories (e.g., data governance, data quality, data architecture). Dividing the broad idea of organizational maturity into themes and dimensions makes it easier for data leaders to identify concrete areas for improvement.
- Consistency: This is key to models being more or less applicable to other organizations within a given industry, and comparative to analogs across industries.
- Comprehensive analysis: Measuring both themes and dimensions ensure that modelers account for all relevant organizational factors—resource allocation, tech infrastructure, data lifecycle management, etc.—while teams measure their organization’s overall data maturity.
Benefits of using a data maturity model
Because these key components make maturity models universally applicable, organizations tend to experience the same general benefits from their use.
Enhanced data quality: Firstly, organizations that endeavor to continuously improve data quality produce more reliable and actionable insights. Moreover, this enhanced data quality fosters better customer service, decision-making accuracy, and better business strategies.
Improved decision-making: For organizations that want to “walk the walk” with data-driven decision-making, data maturity corresponds to the ability to base business decisions on enhanced, high-quality data (i.e., data that is accurate, reliable, and timely).
This is due to the high-quality company data increasing the potency of business strategy, data analytics, data literacy, and business intelligence (BI).
Operational efficiency: Data-mature organizations operating with streamlined data processes lose less time and effort handling their data, which reduces redundancy. This leads to a valuable trifecta—increased productivity, more effective resource use, and cost savings.
Regulatory compliance: High data maturity ensures an organization’s data management practices meet all regulatory requirements, reducing risks of fines and penalties.
Perhaps even more importantly, consumers are increasingly choosing to give their business to organizations with proven track records of respecting and protecting data.
Competitive advantage: Finally, ultimately, data-mature organizations enjoy an edge over less-advanced competitors.
Mature data practices enable faster, better-informed decisions throughout an organization. Additionally, high data maturity supports the organizational agility needed to consistently respond to market changes, innovate, and meet the needs of their customers.
Pros and cons of popular data maturity models
There are many different data models in use today, each with its unique strengths and weaknesses. This is a good thing, as the multifarious datasets each organization works to harness grow ever larger and more complex.
And more model options mean IT leaders have a much better chance of finding which model will be the most perfect fit for their organization.
That said, comparing the pros and cons of these three popular data maturity models can be quite useful:
The Gartner Data Maturity Model
Gartner’s data maturity model is a five-stage framework designed to help organizations assess and improve their data management and analytics capabilities.
Pros:
- Comprehensive framework: This model covers a wide range of data management and analytics aspects, from initial awareness to full integration into business strategy.
- Business alignment: It emphasizes aligning data initiatives with business objectives, ensuring that data management contributes to strategic goals.
- Guided progression: Gartner serves as a textbook case in providing clear guidance on how organizations should move from one maturity level to the next.
Cons:
- Broadly focused: Its comprehensive nature can make it overwhelming for organizations just starting with data management.
- Less prescriptive: This model provides high-level guidance, which may require organizations to interpret how to apply it to their specific context.
The IBM Data Governance Maturity Model
In contrast, IBM’s data maturity model focuses specifically on data governance in order to help IT leaders assess practices across various domains. The model itself has five levels of maturity and emphasizes the management, quality, and protection of data.
Pros:
- A specialized focus: IBM's maturity model, which focuses on data governance, is highly relevant for organizations that need to improve this key and critical area.
- Highly detailed: This limited focus enables in-depth guidance on governance practices, including data quality, security, and compliance.
- Industry-recognized: Widely respected in the field of data governance, it offers best practices based on IBM’s extensive experience.
Cons:
- Narrower scope: By focusing mainly on governance, IBM’s data maturity model might not fully address broader data management and analytics needs.
- Complexity: The model’s detailed nature can be complex to implement, particularly for organizations with less mature data practices.
The TDWI Data Management Maturity Model
In contrast to IBM’s more narrow focus on governance, the Data Warehousing Institute’s own data maturity model is designed to assess an organization’s data management maturity across six dimensions, including data governance, data integration, and analytics. In doing so, it provides a detailed roadmap for improving data management practices.
Pros:
- Holistic approach: The TDWI model covers multiple dimensions of data management, including governance, integration, and analytics. This provides a balanced view of data maturity.
- Actionable insights: TDWI also offers specific recommendations and best practices for each stage, making it easier for organizations to implement improvements.
- Benchmarking capabilities: This model allows organizations to benchmark their maturity against industry standards.
Cons:
- Resource-intensive: Comprehensive modeling comes at a cost, and the model’s recommendations may require significant resources, especially when organizations find themselves at lower maturity levels.
- Detailed and complex: Additionally, the thorough nature of the TDWI model can be challenging to navigate without expert guidance, particularly for smaller organizations.
Making the most out of all levels of maturity
Regardless of where an organization currently stands in its data maturity journey, each stage offers valuable opportunities for growth and improvement. The key to doing so is to focus on continuous improvement, strategically align data initiatives with business goals, and foster a culture that values data as a critical asset.
In this sense, data contracts become essential aspects of codifying and elevating all levels of organizational maturity. Because, with the right guidance, you can ensure that your data initiatives are aligned with your business strategy and poised for success.
Learn more by joining our product waitlist (while there’s still time!) at Gable.ai.