Among the many controversial concepts in data engineering, data mesh might currently be the most deserving of the title “It’s complicated.”
Introduced by Zhamak Dehghani in 2019, the concept of data mesh aims to address inefficiencies and limitations in centralized data management systems. For this reason, many see this approach to data federation as admirable—especially at the rate modern organizations are vacuuming up data to maintain an edge over their competitors.
As is all too apparent, traditional centralized methods of data management are increasingly ill-equipped to keep pace.
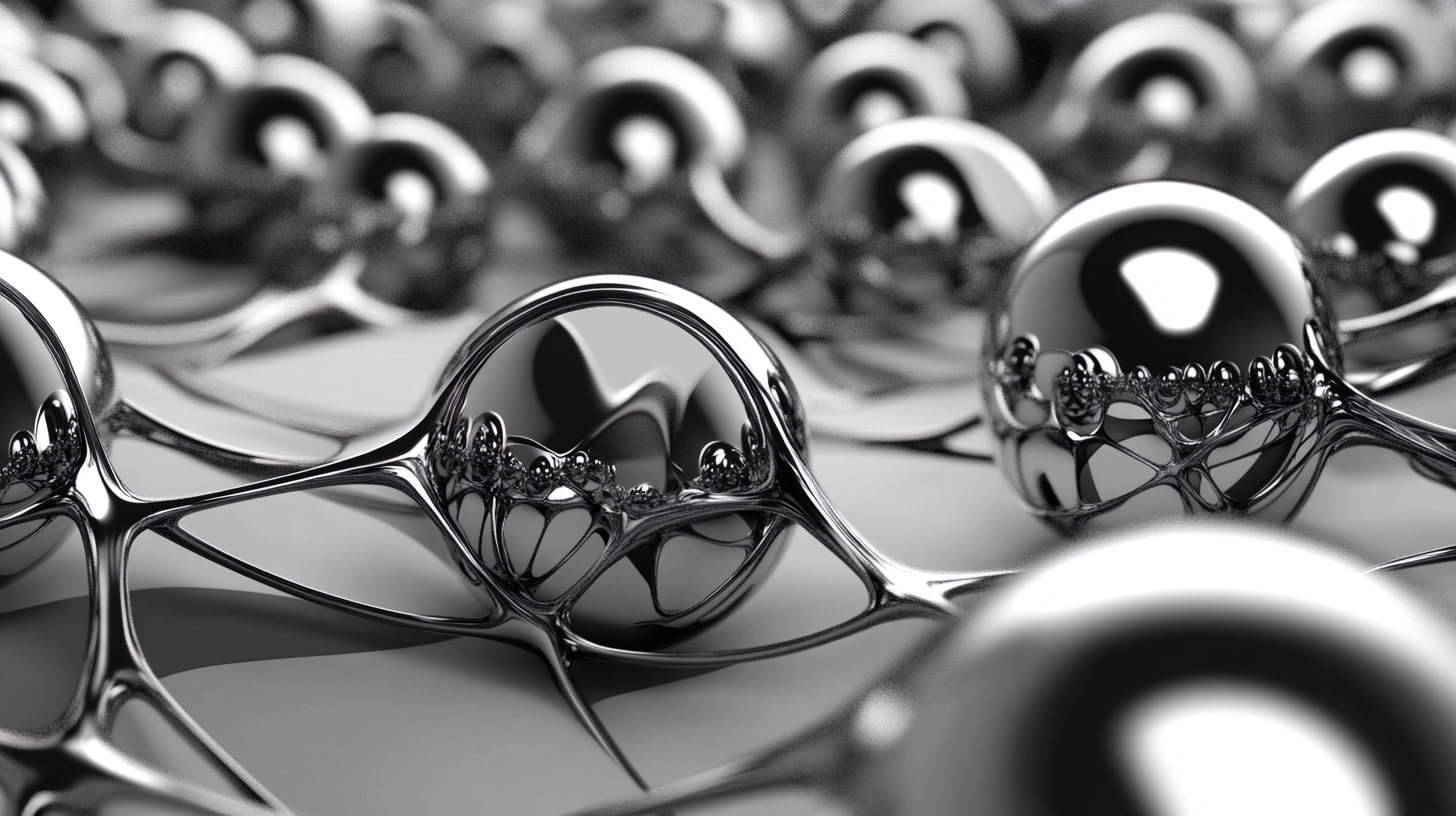
However, what’s been a game changer to some has run afoul to others, as their attempts to solve organizational issues have created more data mesh challenges than solutions.
This begs an important question for data leaders: is data mesh a winning workaround or a wicked problem?
Let’s do the smart thing and break down both sides for analysis to see if we can make more sense of this mess of the mesh.
What is data mesh and why was it created?
Data mesh is a decentralized, self-service approach to data architecture that organizes data around specific business domains—marketing, customer service, and sales are common examples.
Compared to traditional centralized data infrastructures, data mesh shifts the responsibility for data management and governance to domain teams who are better positioned to understand and use their own data products.
In practice, each of these teams then takes responsibility for collecting, transforming, and managing their own data. However, all domain teams bear the added responsibility of treating their data as a product to make the approach viable organization-wide. This means that regardless of department, data teams take it upon themselves to make sure all datasets are easy to consume, maintain clear ownership, and meet all quality and usability standards of their organization.
Like any approach to managing data, benefits vary across organizations and industries. But in general, proponents of data mesh cite four major advantages that it has over traditional, centralized methods of data management.
1. Increased scalability and flexibility
Data mesh architecture enables domain teams to manage their own data products. In doing so, teams can standardize and reuse products across their organization. This freedom facilitates faster scaling while allowing teams more flexibility to adapt to changes. Over time, this allows organizations to scale more efficiently.
2. Enhanced collaboration and innovation
Self-serve data management also bolsters collaboration within organizations, as data democratization is a key operational tenet. This is because, once implemented, data mesh enables teams to discover and use high-quality data for decision-making and experimentation—without relying on a centralized IT team to provide input and access.
3. Faster time to value
This freedom also decouples domain teams from lengthy approval processes that too often hinder innovation in large organizations. As a result, teams can iterate quickly during development cycles, unlocking insights from data products in smaller time frames, which can quickly become a competitive advantage in a rapidly changing business environment.
4. Improved data quality
Arguably, the most important competitive advantage of all is the net increase in data quality that data mesh contributes to. This is due to how self-service data models encourage teams to see and use their data as a valuable commodity as opposed to byproducts of the business’s processes.
What’s more, data mesh encourages domain teams to contribute their own subject matter expertise to their data through their governance of it. This promotes a more holistic lifecycle approach that fosters long-term value and usability.
Additionally, data mesh can solve specific issues that data leaders face—especially in industries that require more specialized workforces and higher levels of data compliance and regulation.
Data mesh over centralization: Key benefits for data leaders
While centralized data architectures have long been the standard, they can struggle to meet the demands of modern organizations.
As businesses grow and evolve, the limitations of centralization—like bottlenecks, data silos, and a lack of domain expertise—can hinder operational efficiency and innovation.
Lack of domain expertise
Centralized teams may lack the detailed domain knowledge required to manage data effectively for specific business needs.
This is especially true in highly specialized industries with unique complexities, like finance and healthcare, where centralized teams often lack the detailed domain knowledge necessary to effectively manage and use data for specific business needs.
However, when data mesh allows teams to organize data around business domains, it empowers domain experts to take ownership of their data—ensuring it is managed and used in ways most relevant and valuable to their specific objectives.
Bottlenecks
One of the most notable issues with traditional data architecture is how it often relies on centralized data teams and platforms (like data lakes or warehouses). Even when managed adamantly, this centralization will be prone to enabling bottlenecks.
This is because as an organization begins to grow, business units need improved data access over time to support increased coordination and communication needs. However, centralized systems can’t scale gracefully as operational demand ratchets up. As such, departments begin to struggle as data access suffers from bottlenecking.
Alternatively, data leaders can leverage data mesh to avoid bottlenecking by assigning ownership of data to domain-specific teams. This way, there are no bottlenecks as domain teams manage their own data as a product, thus reducing or eliminating reliance on a central team for data access needs.
Poor operational agility
Data itself grows more complex and nuanced as organizations grow larger. Centralized data platforms can only adapt so much before new requirements call for extensive re-engineering to keep pace.
Domain-oriented approaches, designed as scalable frameworks, sidestep these overhaul issues and provide organizations with the agility to continuously respond to changing business environments and technological advancements.
Data silos
In many organizations that have undergone extensive growth, it’s common for data to become siloed as different departments adopt new tools and procedures to address immediate needs, rather than big-picture data management cohesion (understandably so). Organizational growth also commonly involves the creation of new business units or departments that each use potentially incompatible systems and practices.
Ironically, the data mesh “divide and conquer” decentralization approach can actively counter this siloing, as stakeholders typically establish a central registry or catalog that registers all domain-owned data products. Post-implementation, this registry allows other teams to easily discover and access data across domains without barriers.
All together, this makes data mesh seem like an exceptional solution for modern organizations. Why, then, do others view data mesh as more trouble than it’s worth?
Four specific shortcomings: Why some data leaders feel the mesh is more trouble than it’s worth
Depending on specific use cases, no approach to data management is perfect. However, understanding the shortcomings and challenges of data mesh is vital for parsing “less than perfect” from “far too problematic.”
First, let’s look at some straightforward aspects that data leaders should be aware of before digging into the most complex challenges inherent in data mesh.
High transformation costs
When transitioning away from centralized data management, data managers should expect data mesh implementation to be both costly and resource-intensive. In addition to establishing decentralized ownership and building self-service infrastructure, leaders must enable change management to create lasting cultural shifts within their organization. While this is entirely possible, it’s never entirely easy.
The idea of owning their own data can be a challenge for some data teams. They may also need to accept and undergo training and upskilling in order to own their own data products and implement governance practices.
Risks of reintroducing data silos
As noted, a core principle of data mesh is that data products should be interoperable and adhere to global standards. However, not all data teams within a given organization may put this core principle into practice. Ironically, in these situations, a data mesh infrastructure can lead to new data silos even as it helps deconstruct traditional ones.
Poor governance and inadequate communication between domains can also contribute to siloed information post-implementation, as data leaders and stakeholders still require centralized coordination and oversight to keep decentralization from devolving into data fragmentation.
Increased complexities in data management
In addition to change management, leadership that’s implementing data mesh is also signing up for more demanding data management overall. At the outset, data leaders must oversee the creation of the self-serve platforms their domain teams will use. They must also manage the newly federated governance their org will rely on while ensuring that communication between the distributed components of the mesh itself is as seamless as possible.
While domain teams work to maximize data quality, it’s the responsibility of leadership to ensure said actual quality manifests. This requires their working with multiple teams that will naturally have varying priorities and expertise.
Misaligned changes by one team can lead to downstream issues for data consumers, such as broken dashboards or incorrect analytics.
Ownership and data governance challenges
If the herding of data quality cats wasn’t enough, determining clear ownership boundaries can be difficult for organizations using data mesh architecture. Competing business priorities and unclear responsibilities can exacerbate these issues, leading to confusion and inefficiencies in governance.
These muddied waters can lead to data quality issues or compound those that may already exist. But data leaders may also face additional critical issues due to ownership ambiguity, including accountability gaps, duplication of efforts (or datasets) between product owners, operational inefficiencies, increased security risks, additional overhead, and organization-wide trust issues relating to the accuracy and value of data in general.
In summary, while data mesh offers significant benefits in terms of scalability, flexibility, and improved data quality, it also presents challenges related to cost, complexity, and governance. Organizations considering this architecture must weigh these factors carefully and plan thoroughly to ensure successful implementation.
Min-maxing: How data contracts can help teams maximize data mesh ROI
For some, debating the principles of data mesh can make for an enjoyable afternoon on LinkedIn. Others, however, are actively down in the trenches, endeavoring to make data mesh deliver for their organization (or will soon join those who are).
These individuals need the data mesh concept to deliver and can’t submit to pain points we’ve mentioned here—such as governance gaps, ownership clarity, and data quality issues. This is why these individuals should realize that, by properly drafting and implementing a data contract, they can alleviate most of the mess inherent in modern-day meshing.
To clarify, let’s directly address each of the four common shortcomings above to provide a sense of just how much help a singular data contract can be:
1. High transformation costs
Data contracts can reduce transformation costs by clearly defining the expectations for data quality, structure, and semantics between data producers and consumers. This minimizes the need for extensive downstream transformations.
By enforcing schema consistency and providing guarantees about data formats and delivery, data contracts ensure that data is ready for use without requiring additional processing. Those who take this proactive approach can avoid costly reworks caused by any schema changes or quality issues downstream.
2. Data silos
Despite being a common challenge in larger organizations, data contracts mitigate data siloing by fostering interoperability and standardization across all domains and defining clear interfaces for data sharing. In this sense, they supplant the manual needs of domain teams to ensure that their data products are discoverable, self-describing, and accessible across the organization.
Moreover, data contracts promote shared metadata and governance standards, which enhance cross-domain collaboration and prevent dataset isolation.
3. Added complexity to data management
We can’t avoid any of the complexities data mesh introduces to data management. However, due to the formalization between data sources and consumers, data contracts keep data management of a meshed ecosystem from being more complicated than it needs to be.
Contracts also provide transparency into dependencies and usage patterns, streamlining data pipeline management and troubleshooting.
Data leaders can benefit further by embedding governance policies directly into their data contracts. By doing so, they can automate compliance checks and reduce manual oversight.
4. Data ownership and governance challenges
Finally, a well-drafted data contract will address ownership and governance challenges by explicitly defining roles, responsibilities, and usage policies for each data product in the organization. They naturally align with the federated governance model of data mesh by balancing domain autonomy with centralized oversight.
Contracts also enforce accountability for data quality and compliance, making it easier to manage regulatory requirements and audits.
Clearly, a singular data contract can transform what some see as a wicked problem into advantageous architecture. While this may not solve the great debate around the viability of data mesh itself, contracts can certainly benefit those in organizations who’ve already opted to decentralize their data fabric.
Fully realize your own data mesh success
Integrating data contracts into your data mesh strategy can be more than a safeguard—it’s also a multiplier of ROI.
These contracts bridge the gap between decentralized data ownership and centralized governance, enabling domain teams to operate autonomously while adhering to organization-wide standards.
By fostering accountability, improving data quality, and reducing silos, data contracts empower VPs of data to transform challenges into opportunities. And if that sounds like a great idea, learn more about making it actionable by signing up for our product waitlist at Gable.ai.