Data management continues to evolve at a rapid pace, underscoring the need for data teams to maintain optimal data quality within their organizations. To do so, these data professionals rely more on data quality tools—sophisticated software applications— to foster and maintain exceptional data quality throughout its lifecycle.

But the question of which tool to turn to quickly gets interesting. One industry's "best data quality tool" may barely cut the proverbial mustard in another. Therefore, it's worth clearly defining what data quality tools consist of before comparing and contrasting your options to understand better what makes each one valuable.
What is a data quality tool?
Data quality tools are software applications or suites that ensure the accuracy, completeness, and reliability of data. Often used by data engineers within an organization, they automate and streamline the processes of identifying, understanding, and correcting flaws in data.
Such tools are indispensable in data engineering, enabling organizations to leverage their data assets effectively. At the same time, they support better decision-making, operational efficiency, regulatory compliance, and (increasingly) the successful deployment of advanced data analytics and machine learning models.
4 popular data quality tools
Fortunately, data engineers can choose from a wider array of data quality solutions than ever. This is thanks to the growing emphasis on data-driven decision-making and the increasing complexity of IT ecosystems.
The best data quality tools range from open-source projects to enterprise-level solutions. Each has unique features, integration capabilities, and support ecosystems to cater to different needs, scales, and complexities of data environments.
While far from comprehensive, the following list of three popular data quality management tools in use today showcases their similarities and differences:
1. Informatica
Informatica offers many features designed to help organizations ensure optimal data quality.
Its data quality tools are part of its broader data management offerings, which include solutions for data integration, data governance, master data management, and more.
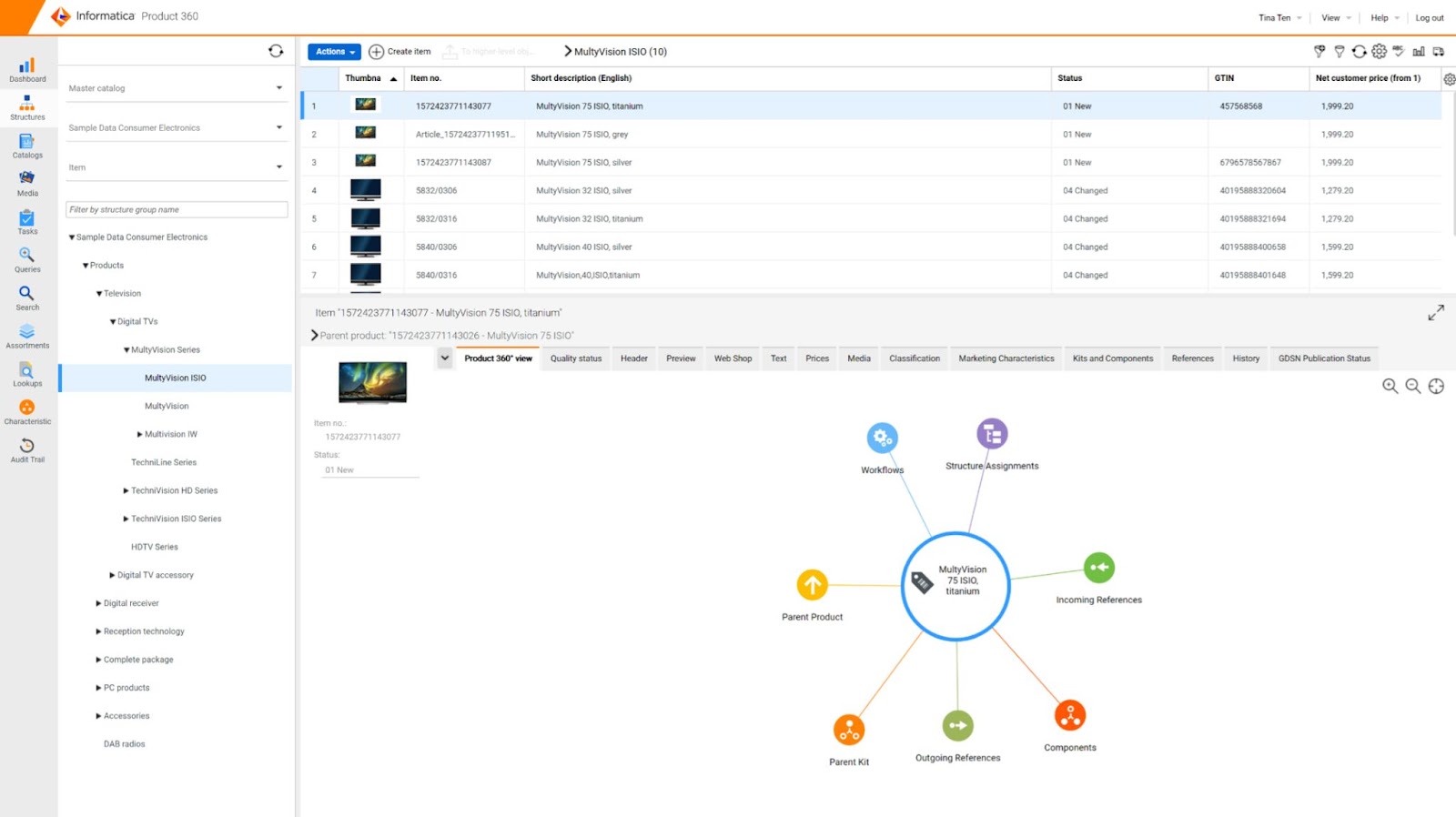
Pros:
- Comprehensive data quality management: Informatica Data Quality (IDQ) provides a wide range of functionalities that allow users to profile, cleanse, standardize, and enrich data. It also supports data governance and compliance efforts and provides a platform for high-quality data.
- User-friendly interface: IDQ features a user-friendly drag-and-drop interface that simplifies the creation and management of data quality rules and processes, making it accessible not only to technical users but also to business users.
- Informatica Analyst: Informatica Analyst’s friendly user interface (UI) enables users without deep technical knowledge to access data quality metrics and functions easily.
- Integration capabilities: Informatica’s tools integrate with various data sources and applications, which is essential for organizations to optimize data quality processes in diverse data ecosystems.
- Scalability: Informatica’s solutions are scalable, cater to the needs of large enterprises, and can handle significant volumes of data.
- AI-powered: With the CLAIRE™ engine, Informatica automates critical tasks such as data discovery, which enhances productivity and efficiency.
Cons:
- Cost: Informatica may be expensive compared to other data management tools, which could make it prohibitive for smaller organizations and/or those with limited budgets.
- Complexity and resource intensiveness: Some users find Informatica’s tools challenging to learn, and the tools can be resource-intensive in terms of hardware and infrastructure requirements.
- Outdated user interface: Although powerful, the user interface is somewhat dated and needs modernization.
- Technical support: Reports of slow and sometimes unresponsive technical support could be a concern for organizations that require prompt assistance.
- Integration challenges: While Informatica offers strong integration capabilities, some users have reported difficulties integrating with third-party applications.
Pricing: Informatica doesn’t share pricing details on its website, so interested parties have to contact the company for a customized quote.
2. IBM InfoSphere
IBM InfoSphere is a comprehensive set of data integration and data quality software products for achieving data accuracy, completeness, and reliability across various systems.
Infosphere enables profiling, cleansing, matching, and monitoring. All four are essential for maintaining high-quality data for business intelligence, data warehousing, application migration, and master data management projects.

Pros:
- End-to-end data quality management: InfoSphere offers a full range of tools to help data professionals understand data relationships, monitor quality, and cleanse, standardize, and match data.
- IBM Databand: A recent acquisition, IBM's data observability platform supports InfoSphere users, offering end-to-end data lineage and impact analysis solutions.
- Flexible deployment options: InfoSphere tools also support deployment on-premises, in the cloud, or both, offering flexibility to organizations that have varying IT infrastructure preferences.
- Industry-specific solutions: With multiple editions and models that support a range of industries, data professionals can adapt InfoSphere to a variety of business needs.
- Advanced data governance: The platform includes features for data governance (such as data profiling and cleansing), metadata management, and data lineage tracking.
- Ability to scale: InfoSphere’s data quality tool can also handle complex integration tasks and large volumes of data, making it suitable for enterprises of all sizes.
- Data catalog and data discovery capabilities: This functionality can specifically benefit robust data provenance systems within an organization.
Cons:
- Costs: Like Informatica, InfoSphere is more expensive than some other data quality solutions. This could make it a non-starter for small, new, or budget-constrained businesses.
- Complexity: The complete InfoSphere suite is comprehensive—meaning less-experienced data professionals may face a significant learning curve.
- Implementation: The initial setup and implementation process can be complex and may require IBM support and expertise.
- Customizability: Some users may find InfoSphere's data quality tools less flexible and customizable than other tools on the market.
- Dependence on vendor support: Organizations lacking a robust data talent roster may become dependent on InfoSphere’s parent company, IBM, for support and expertise.
Pricing: IBM InfoSphere follows a subscription-based model, with costs depending on the number of users, the level of support required, and the functionalities needed by the organization.
Additionally, IBM has consumption-based pricing models. The Informatica Processing Unit (IPU) is one example and allows organizations to pre-pay for usage on an annual basis and provides the flexibility to use a range of cloud services.
3. Ataccama ONE
Ataccama ONE is a self-driving data management and governance platform that includes data quality among its core functionalities.
The platform aims to provide a unified approach to managing data quality, governance, and master data management. It uses AI to automate and enhance data quality processes.
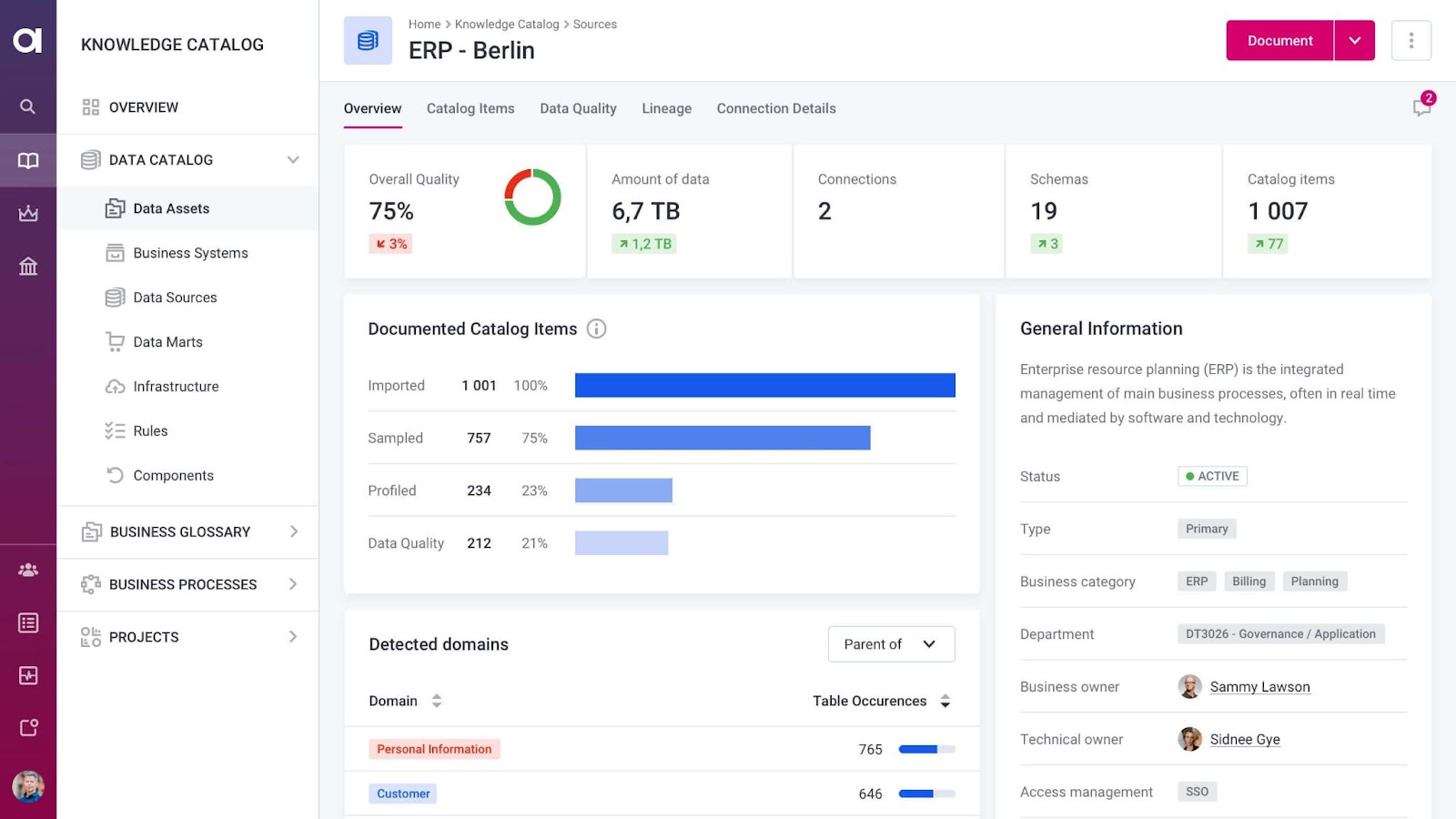
Pros:
- Free data profiling: Ataccama ONE offers free data profiling—a valuable feature for understanding data sources and improving data quality.
- AI-enhanced data quality tools: The platform also includes AI-powered features that enhance data quality tasks such as anomaly detection and automated rules assignment.
- Ease of integrations: Users appreciate how they can integrate Ataccama ONE with other systems for complete end-to-end data management and governance.
- Runs natively on big data platforms: Ataccama ONE can run natively on the most common big data platforms (e.g., HDFS, AWS, Apache Spark, GCP), which can benefit data teams working with large datasets.
- Responsive Customer Support: Some users report that Ataccama provides comparatively responsive customer support.
Cons:
- Complexity: Ataccama ONE can be complex to learn and may require training, especially for less technical users
- Implementation: The implementation process can be lengthy and complicated, which could pose a challenge for some organizations
- Automation limitation: Some users feel Ataccama ONE has limited automation options, potentially affecting efficiency
Pricing: According to details noted in its forums, Ataccama's pricing model is based on two main factors: the type of user and the size of the processing engine.
Users fall into two groups: those who manage and author the data (like administrators and data stewards) and those who only view the final results. Subsequent costs vary depending on their roles and the modules they use. The price for the processing engine depends on the number of cores needed to handle the data efficiently, which is determined by factors like the complexity of operations and the amount of data processed.
At this time, Ataccama doesn't charge per record or source but provides a questionnaire to help estimate the right engine size for each customer's needs.
4. Gable.ai: “A new data quality tool has entered the chat!”
Gable.ai's first data contract tool will soon be available to data professionals. Like Informatica, InfoSphere, and Ataccama, our data contract tool will offer a robust new way to ensure optimal data quality at the very beginning of the data lifecycle:
Our tool enables data professionals to integrate data contract functionality directly into their respective software development workflows. As such, Gable.ai will offer specific advantages compared to other data quality tools currently available.

Pros:
- Automated data contract enforcement: Gable.ai provides real-time data validation, enforcing data contracts through CI/CD pipelines. Once integrated, any changes to data or its structure are checked immediately against established contracts. This provides instant feedback to data teams and prevents violations from progressing further along in the development cycle.
- Proactive data quality management: This enables data teams to manage data quality proactively, instead of rushing to fix data quality issues after they've occurred. In this way, Gable.ai maintains data integrity throughout the development process, reducing costs and wasted effort as a result.
- Developer-friendly interface: We've designed Gable.ai to integrate seamlessly with development environments—particularly with version control systems like GitHub, allowing data contract enforcement to function as part of the coding process itself.
- Enhanced collaboration and transparency: The tool will enhance collaboration by notifying relevant stakeholders directly within a given development platform. These alerts, detailing potential violations and required actions, help align developers, data scientists, and business analysts around data quality standards and compliance.
- Customizable responses to data contract violations: Additionally, Gable.ai allows organizations to configure the fidelity of how violations are handled, tailoring data contract enforcement to their risk tolerance and unique operational needs. Configuration options include warnings (soft stops that notify but do not block changes) and hard stops that prevent merging until issues get resolved.
Pricing: If you are interested in learning more about potential pricing models for Gable.ai, sign up for our product waitlist.
Criteria to consider when choosing data quality tools
No matter the platform or tool they’re vetting, data engineers should consider a holistic set of criteria to settle on one that aligns with their organization’s data quality needs, technical capabilities, and business objectives.
While you must account for unique characteristics of a given organization, there are some important criteria for vetting the best data quality management tools. Here are the top capabilities to look for:
- Data quality functions: Potential tools should support essential data quality functions such as data profiling, data cleansing, data parsing, matching, and enrichment.
- Service-oriented architecture (SOA) integration: A good data quality tool can package data quality rules and workflow into a service that can be invoked via SOA calls, allowing for seamless integration with other systems.
- Rules engine: Robust rules engines are important for ensuring data quality rules are created, managed, and invoked. Potential tools should support the packaging of rules into a library and allow for their invocation via SQL or other languages.
- Reports and business intelligence (BI): Tools should offer out-of-the-box reports and BI capabilities, supported by the definition of data quality thresholds and tolerances, or allow access to the backend data quality store.
- Metadata support: Metadata is crucial for understanding the data’s lineage and context, so the tool should offer strong metadata support.
- Batch processing capabilities: The ability to schedule regular data cleaning practices is helpful for maintaining ongoing data quality.
- Self-service capabilities: The tool should be simple for everyone on the team to use, including the ability to set up dashboards and share insights.
- Collaborative features: Data quality tools should facilitate collaboration, allowing team members to share data, insights, dashboards, and reports easily.
- Automatability: Contenders should allow for the automation of the monitoring process and workflow, reducing the need for manual involvement.
- Privacy: Any best-in-class data monitoring tool needs to provide security leverage against external threats and ensure compliance with data protection regulations.
Additionally, there are several other things worth considering:
- Licensing model: Understanding the licensing model for any data quality tool is crucial, as it affects the overall cost. Consider whether pricing is per seat, enterprise-wide, or offers a freemium model with basic functions at no cost and a fee for more advanced options.
- Performance and scalability: Assess the tool’s performance benchmarks for processing a large number of data quality rules or profiling data, as well as its support for a number of concurrent users.
- Product architecture: Evaluate product architecture, especially as it relates to application tiers, metadata acquisition, integration, and deployment options.
- Data types supported: Any tool making the shortlist should support a wide variety of data types, including unstructured, semi-structured, and structured data. Note: Native Hadoop support may also be a factor if it is important for the organization.
- Ease of deployment and installed base: Consider ease of deployment and management for a tool, as well as the number and types of installations in production.
- Product roadmap: Finally, understanding the future functionality planned for the tool can provide insight into the product’s maturity and the sophistication of the product management team.
Investing in the complete data lifecycle
As our sampling above shows, data engineers have a plethora of top data quality tools. Each has its own strengths, weaknesses, and unique features designed to cater to various needs, scales, and complexities of data environments. From comprehensive enterprise solutions like Informatica and IBM InfoSphere to open-source platforms like Talend, these tools automate and streamline the processes involved in maintaining data quality and understanding data.
One way to ensure quality throughout all phases of the data lifecycle is through the implementation of data contracts. These define clear standards and expectations—from collection to consumption—for data quality, format, and use between data providers and consumers.
Curious to learn more about data contracts? Be sure to sign up for our product waitlist at Gable.ai!
This article is part of our blog at Gable.ai — where our goal is to create a shared data culture of collaboration, accountability, quality, and governance.
Currently, our industry-first approach to drafting and implementing data contracts is in private Beta.
Curious to learn more? Join the product waitlist to be notified when we launch.