It’s not surprising that those in the financial sector (banks specifically) are chompin’ at the proverbial bit to cash in on the benefits of big data.
According to a recent survey of investment bank (IB) stakeholders in FICC trading, sales, and data management—in addition to analysis of data sourced from global investment banks tiers 1 to 3)—83% of banks say they want access to real-time analytics capabilities.
However, financial institutions also have a bit of a problem.
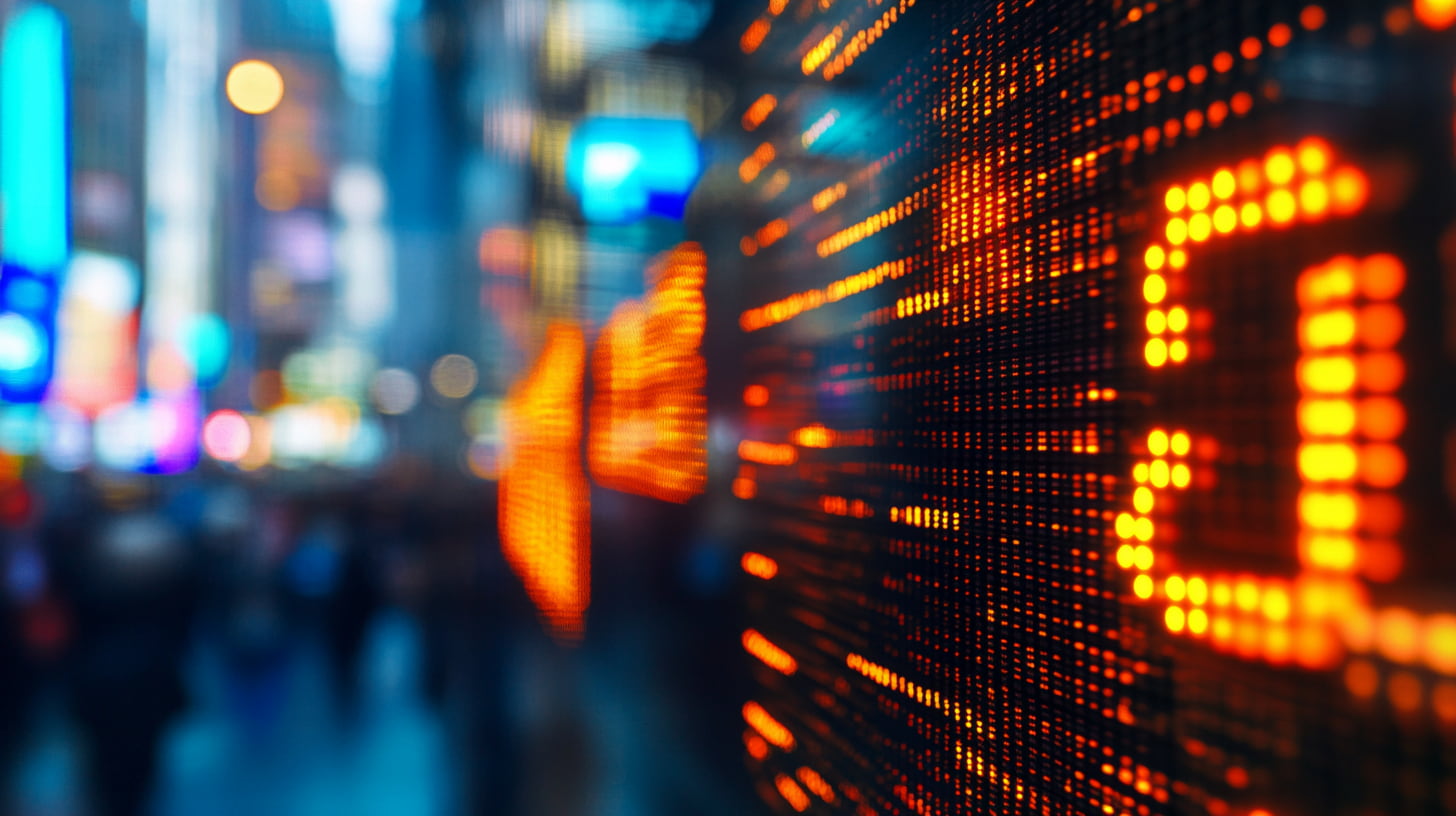
According to Gartner, organizations in the financial sector experience an average annual loss of $15 million due to poor data quality. This substantial financial impact underscores the critical need for high-quality data to ensure accurate decision-making and efficient operations.
A survey by Mosaic Smart Data revealed that 66% of banks struggle with data quality and integrity issues. These challenges include gaps in important data points and incomplete transaction flows, which hinder the ability to effectively use AI-powered analytics for improving efficiency and profitability.
The same survey found that 83% of banks lack real-time access to transaction data and analytics, primarily due to fragmented data systems and the absence of a central repository. This limitation significantly affects banks and their ability to leverage data for timely and strategic decision-making.
But there’s good news on the horizon. As more data professionals embrace the intricacies of financial data quality issues, the best solutions to these issues are becoming more and more apparent.
However, the road to this good news needs to begin at the beginning—by clearly defining financial data quality.
What is financial data quality?
Data quality, on the whole, often refers to the combined accuracy, consistency, and reliability of data used by a given organization. But this useful, catch-all definition can (and should) vary depending on which industry said organization operates in.
As such, the quality of data used by financial institutions for decision-making may be measured by its accuracy, completeness, and timeliness. These factors have a greater impact on the ability of those in the finance industry to make sound financial decisions. Therefore, maintaining data integrity is crucial in financial systems.
When financial data is of high quality, it is free from errors, redundancies, and inconsistencies, which ensures that all processes relying on that data are efficient. For example, accurate data in a financial report enables more precise forecasting and risk management.
On the other hand, poor data quality can disrupt operations, cause financial losses, and expose organizations to regulatory penalties. Decision-makers in financial institutions rely heavily on high-quality financial data to assess market trends, customer behavior, and investment risks. Ensuring the integrity of this data means ensuring the organization's decisions are based on reliable, accurate data sets.
As such, financial data quality is an essential subset of overall data quality and plays a significant role in ensuring sound decision-making in financial institutions—as long as the specific needs of the financial industry are met.
The unique challenges of data quality for financial institutions
Financial institutions face several unique challenges when addressing data quality issues, given the complexity of their operations, stringent regulations, and the critical nature of the data they handle. Let’s walk through some of the most prominent challenges.
Data format variety
Financial institutions collect and process data from multiple sources, such as transactions, customer information, and market data.
These come in various formats, including structured data like spreadsheets and unstructured data such as emails or PDFs. Ensuring consistency and accuracy across these disparate formats is a key challenge, as discrepancies in formats can lead to significant data quality issues.
Regulatory compliance
Financial institutions are subject to strict regulations, such as the Basel Accords, GDPR, and local financial reporting standards. Maintaining high data quality is essential for regulatory compliance, as errors or missing data could result in fines or sanctions.
Institutions need to ensure their data is accurate, timely, and complete to meet these legal requirements, which can be difficult when dealing with complex datasets and frequent regulatory changes.
Data discrepancies
Inconsistent data across different systems or departments often leads to issues that hinder decision-making. For example, a customer’s account details might be stored differently in different departments, causing reconciliation issues. Such discrepancies can create major inefficiencies and potential financial risks.
Risk management
Effective risk management depends on high-quality data for accurate assessments of market trends, credit risks, and customer behaviors. Data quality issues can undermine the integrity of risk models, leading to incorrect conclusions and poor decision-making, which may increase the institution's exposure to financial risks.
That said, while these challenges are unique, there are also common issues that should not be discounted.
Common data quality issues faced by financial institutions
Financial institutions commonly face several data quality issues that can impact their operations, regulatory compliance, and decision-making processes. Here are some of the most frequent of these challenges.
Poor data quality
Financial institutions often deal with poor data quality in the form of incomplete, inconsistent, or inaccurate data. This can result from legacy systems, manual data entry errors, or integration issues between different systems.
Poor data quality can have serious consequences for regulatory compliance, risk management, and operational efficiency.
Data validation
Ensuring that data is correctly validated before being used is a critical challenge. Many institutions face issues with insufficient validation checks, which leads to incorrect data entering critical systems.
Without robust validation processes, errors can propagate through the system, affecting everything from customer records to financial reporting and decision-making.
Audits and regulatory requirements
Financial institutions are subject to frequent audits and regulatory scrutiny. Ensuring that data is of high quality is essential for meeting these requirements, but poor data quality can result in failed audits, penalties, or sanctions. Accurate and complete data is needed for auditing purposes, particularly in areas like financial reporting, where errors can lead to significant regulatory consequences.
Data governance
A well-established data governance framework is vital for maintaining high data quality, yet many institutions struggle with implementing effective governance practices. Without proper data governance, institutions may lack clear ownership, responsibility, and processes for managing data, resulting in inconsistent data handling and poor-quality outputs.
Financial reporting
Financial reporting is another area where poor data quality can cause significant issues. Inaccurate or incomplete data can lead to incorrect financial statements, misinforming stakeholders, and potentially causing regulatory breaches. Financial institutions must ensure that the data feeding into their reporting systems is accurate and validated to avoid these risks.
Tips for managing data quality in financial institutions
Managing data quality in financial institutions requires a proactive and structured approach due to the critical nature of financial data. Here are key tips for effectively managing data quality:
Implement a robust data governance framework:
- Establishing a comprehensive data governance framework is essential for maintaining high data quality. This framework should clearly define roles and responsibilities for data management, ensuring accountability and oversight across all departments.
- By enforcing consistent data standards, data governance helps prevent issues such as data duplication, inaccuracies, and inconsistencies, ensuring all data is reliable.
Strengthen data validation processes:
- Implementing strict data validation checks at every stage of the data lifecycle is crucial. Financial institutions should ensure that data entering their systems is accurate, complete, and meets predefined quality standards.
- By automating data validation rules, institutions can minimize manual errors and ensure that only high-quality data is used for decision-making, reporting, and risk assessments.
Utilize real-time data management solutions:
- Financial institutions deal with large volumes of data that need to be processed quickly. Adopting real-time data quality tools helps ensure that data is up-to-date and accurate across systems.
- Real-time data also allows institutions to respond swiftly to market changes, monitor transactions, and ensure regulatory compliance, all of which depend on having high-quality, timely data.
Increase data observability:
- Data observability refers to the ability to continuously monitor and assess data quality across systems. Implementing observability tools helps financial institutions detect issues like data anomalies, missing data, or discrepancies in real time, allowing for faster resolution.
- This ongoing monitoring ensures that data quality remains high, even as new data is ingested or systems change.
Regularly audit and refine data management practices:
- Conduct regular audits to assess the effectiveness of your data management and quality controls. By frequently reviewing and refining these practices, financial institutions can identify emerging data quality issues early and adapt to changes in regulations, technology, or internal processes.
- As a whole, focusing on a strong data managements framework, effective data validation, real-time data solutions, improved data observability, and regular audits, financial institutions can significantly improve their data quality and maintain reliable, accurate data for decision-making.
The way forward: Enhancing data quality for financial success
It’s now clear that the importance of data quality in financial organizations cannot be overstated. As stakeholders continue to demand accurate, real-time analytics, institutions must prioritize robust data management strategies to stay competitive and compliant.
Addressing challenges like fragmented data systems, regulatory requirements, and poor data governance is critical to ensuring reliable decision-making, risk management, and financial reporting.
To learn more about how high-quality data can transform your financial operations and improve stakeholder confidence, sign up for our product waitlist today and be at the forefront of financial data innovation.